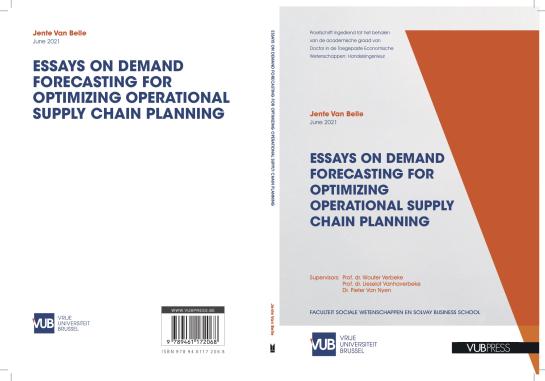
High quality short-term demand forecasts are a crucial input for optimizing operational supply chain planning. This PhD thesis mainly focuses on the generation of such high quality inputs by pursuing improvements in short-term demand forecasting. However, the link between short-term demand forecasting and operational supply chain planning is also approached from a different angle by considering the supply chain design to align it with demand forecasting capabilities, i.e., so that the forecasting system in place is optimally used.
As to the pursuit of improvements in short-term demand forecasting, this works considers both the use of more diverse data sources (net to historical demand), often referred to as demand sensing solutions in the business forecasting literature, as well as the use of more advanced forecasting algorithms and methodological innovations. As for the former, in this thesis, an empirical study on the use of so-called downstream data to improve the accuracy of forecasting wholesaler demand in multi-echelon supply chains is presented. Regarding the latter, the focus is on extending the literature on global deep learning models for time series forecasting. First, a methodology is proposed to extend the state-of-the-art N-BEATS deep learning architecture to allow optimizing forecasts from both a traditional forecast accuracy perspective as well as from a forecast (in)stability perspective, which is used in this work to refer to variability in forecasts for a specific time period caused by updating the forecast for this time period when new observations become available, i.e., as time passes. For short term demand forecasts which are used as input to operational supply chain planning, next to being highly accurate, being stable is also a desirable characteristic. Second, after presenting a modification to the N-BEATS architecture for generating Gaussian probabilistic forecasts (i.e., a full predictive distribution), the proposed forecast stability mechanism is translated to this probabilistic forecasting setting. This work also presents a second extension to this probabilistic N-BEATS model which is useful in an inventory management context in that also probabilistic forecasts of temporal aggregates (i.e., cumulative demand forecasts) are learned by the network.
Finally, as for approaching the link between short-term demand forecasting and operational supply chain planning by considering changing the supply chain design to align it with demand forecasting capabilities, specifically, a framework that serves as decision support tool for making lead time decisions in a periodic review dynmaic order-up-to inventroy system is proposed.