![]() |
Scope & objectives | News & events | Project Outputs | Contact |
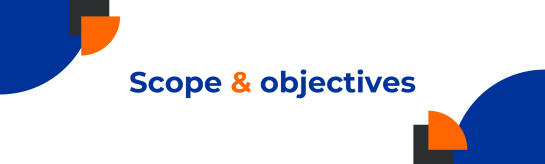
The main essence of the innovation of the MAMûET project is on research enbaling the co-design optimization and the smart management, control and exploitation of a multi-energy microgrid. Accordingly, the facilitation and development of a Cooperative Ecosystem is key inside this project. Inside this collaborative scenario, three main goals have been described for MAMûET:
-
Cost effective exploitation of a microgrid by optimal co-design and real time control of the assets.
-
Optimization of multi-energy management for increased Total System Efficiency.
-
Standardize, Scalable and Replicable Proof of Concept of the Cooperative Eco Platform.
To realize these goals, following main knowledge acquisition is essential:
-
Self-learning algorithms for State estimation of assets and predictive analytics for the integration of a wide variety of connected devices and advanced renewable energy production and storage.
-
Optimal management and control strategies for reliable operation, optimal balancing and stabilization of voltage and frenquency, with maximal use of renewables, supporting fast and reliable off-on-grid transition.
-
Business and exploitation models based on predictions of multi-energy virtual merit orders, enabling beneficial trading of energy within the microgrid and between the microgrid and the grid.
Copyright © 2022
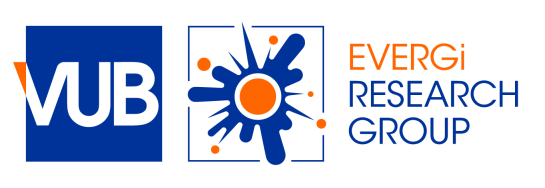
The self-learning, predictive algorithms to be researched in this project will optimize the operational multi-energy management of the microgrid in such a way that prolongs the asset lifespan and exploit the assets' generation and storage capacities. The algorithms will be based on big-data analytics and self-learning approaches. A multi-vector multi-agent system is a complex system that combines different types of devices (such as wind turbines, PV-panels, batteries, electric vehicles,...) from different energy-vectors (such as electricity, heating, cooling, ventilation, water,...). The potential of optimizing such a grid is large considering the array of possible source dispatching and storage capacities in response to different demand types. The self-learning algorithms that will be developed will predict the demand/supply and optimize the co-design (CAPEX reduction estimated up to 30%) as well as the operation and maintenance (OPEX reduction estimated up to 15%). The optimization includes a complex set of external factors such as grid prices, levelized cost of local energy, weather, CO2 taxation and environmental.
The basic is covered by VUB being the academic partner with a proven track record in State and Behavior Predictive Models and design tools for energy systems. The industrial partners: ABB, Power Pulse, Priva and SDM, will ensure innovation technical and financial feasibility. The industrial partners have complementary specializations that ensure project success, motivated by improving their operations and business processes.